Microdata case study: Over-indebtedness in the UK
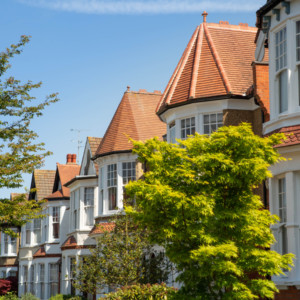
What is microdata and what can we use it for? This is the first blog in a case study series that explains the merits of microdata in policy analysis. This particular blog investigates over-indebtedness in the UK, using the 6th round of the Wealth and Assets Survey published by the UK’s Office for National Statistics (ONS) in 2019.
What is microdata?
Microdata are detailed sets of records taken from sample surveys, censuses and administrative systems which can often be accessed by the public from UK Dataservice. For example, a microdata set for an individual could contain information such as their age, education, religion, and employment status. Such targeted datasets enable researchers to study issues such as over-indebtedness at a detailed scale, generating their own statistics to inform policymaking, or to measure the impact of a targeted policy intervention.
Microdata allows us to go beneath the surface of traditional aggregate statistics to shed light on the circumstances of different groups. They are an increasingly important source of insight for our work here at Cambridge Econometrics.
Case Study example: Measuring debt and over-indebtedness through the Wealth and Asset Survey (WAS) microdata set
The Wealth and Asset Survey (WAS) is a biennial study that measures the well-being of households in terms of their assets, savings, and debt.
During my undergraduate studies at the University of Aberdeen I explored several different aspects of inequality including debt and over-indebtedness. I chose to look at the Wealth and Asset Survey (WAS) as a case study to gain a deeper understanding of not just how many households were affected by over-indebtedness in the UK, but the age, life stage, and geographical area of those affected.
The Survey also provided me the opportunity to grasp the social experience of over-indebtedness as well as the economic reality; one of the main features and benefits of using the Wealth and Asset Survey microdata.
Here I looked specifically at two indicators of over-indebtedness:
- Qualitative indicator: defined by household responses to the WAS questionnaire sharing that they find servicing their debt a heavy burden.
- Quantitative indicator: assesses debt sustainability and is calculated as the ratio of debt repayments to income – when this exceeds 25% the household is characterised as over-indebted.
By using these two indicators, I was not only able to identify a subjective debt measure and debt repayment ratio, but also who is most affected by over-indebtedness.
Key findings
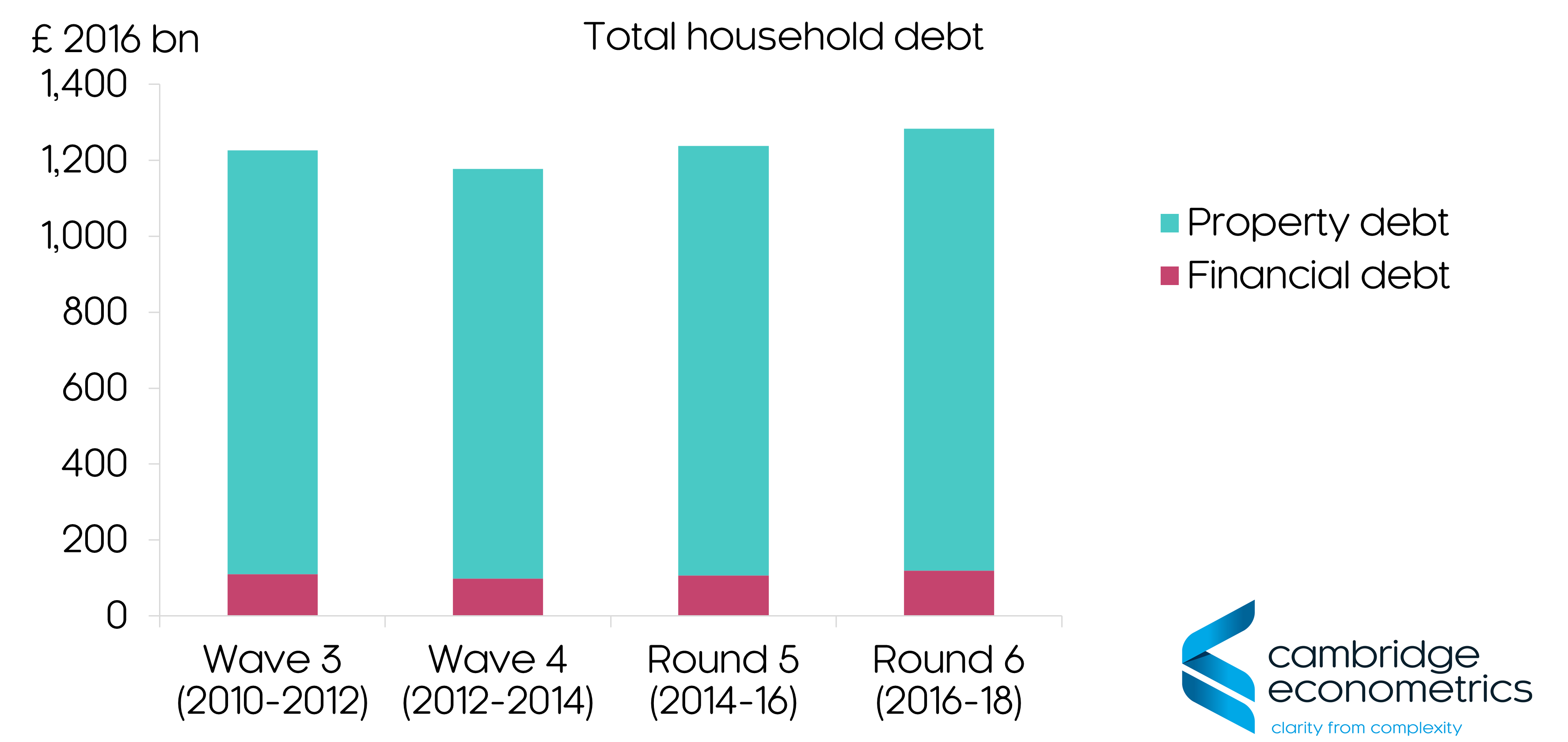
The last four rounds of the Wealth and Asset Survey (WAS) show that, in real terms, household debt has been increasing. However, 95% of the value of all debt is property debt (mortgages and equity release), which is often deemed “good debt” (Chart 1). This is because it represents investments in assets that increase in value. The key findings of the latest WAS show that the trend of increasing property debt has continued:
- Property debt makes up the largest share of total debt in the UK.
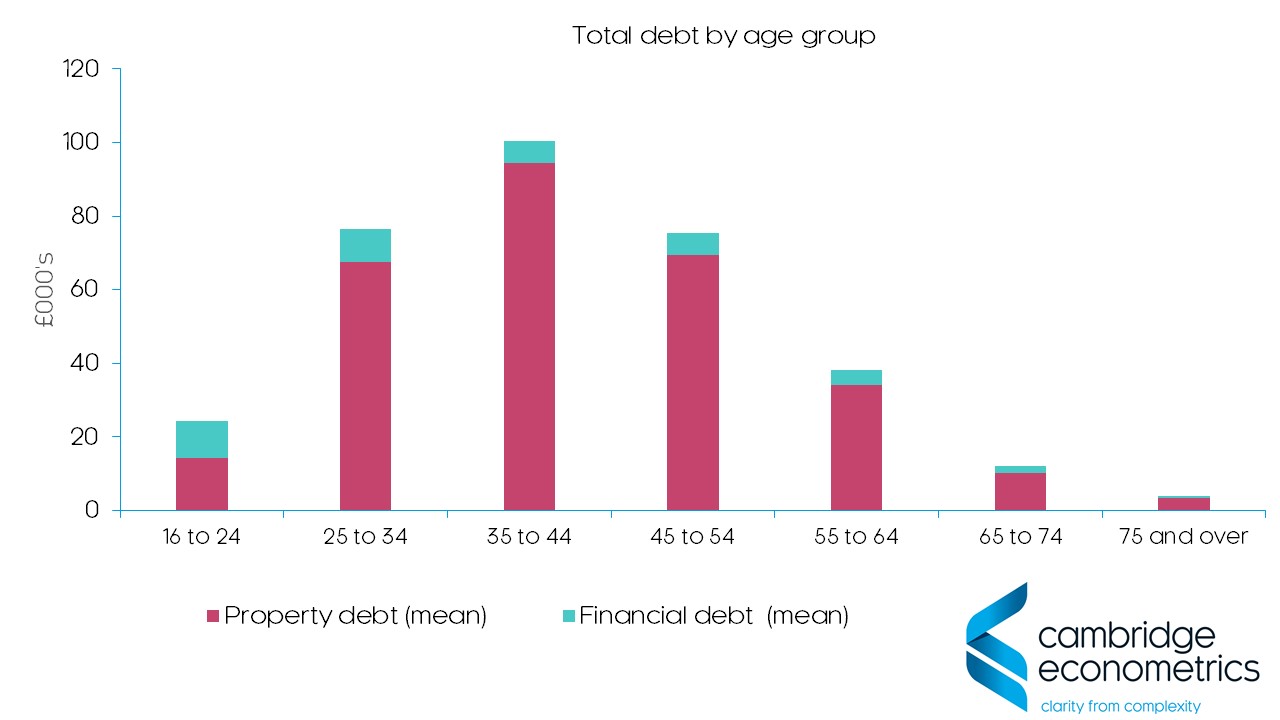
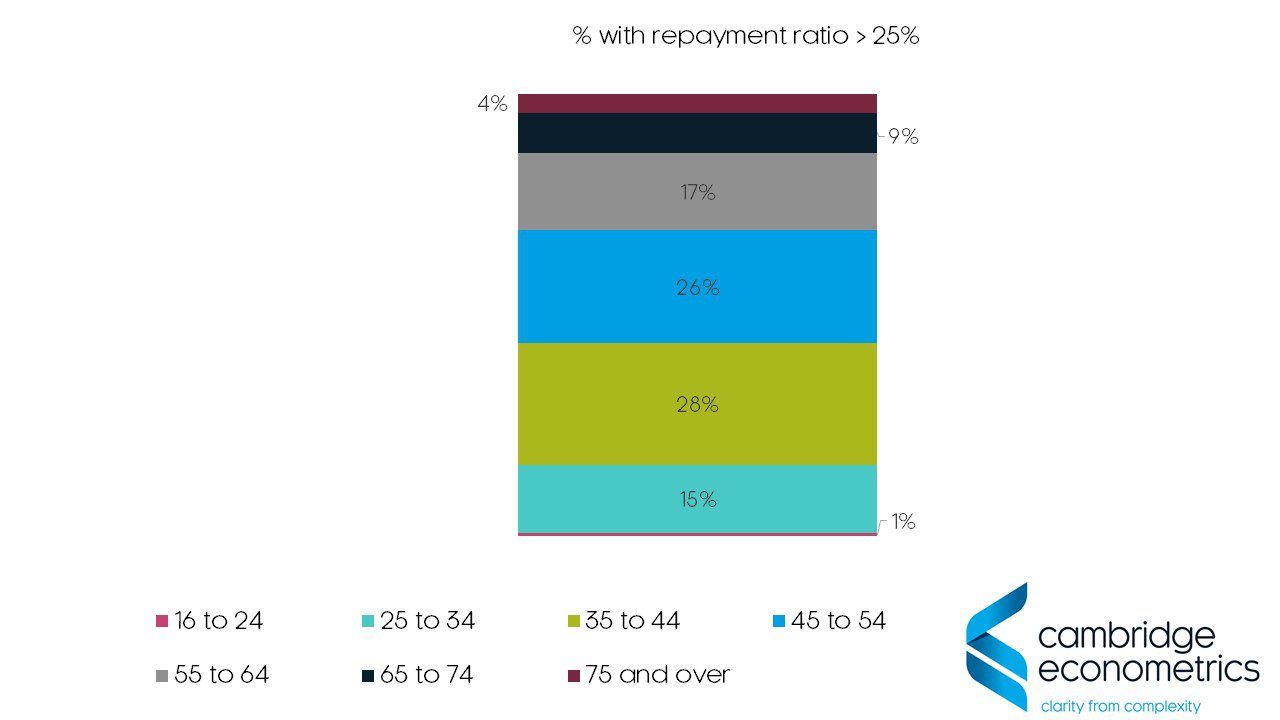
- Age – households led by someone mid-career hold most debt and make up the majority of the over-indebted (Chart 2) and (Chart 3).
- Homeowners – people who own their homes outright are less likely than those still paying off mortgages to be over-indebted.
- Location – London has the highest share of over-indebted households in the UK.
- Wealth decile – the richest households have the highest debt levels, but the poorest hold the most financial debt. The subjective feeling of debt being a burden has decreased from nearly 9% of respondents to 6.8% in the most recent wave of the WAS. The richer the household, the more likely it does not consider debt levels a burden (Chart 4).
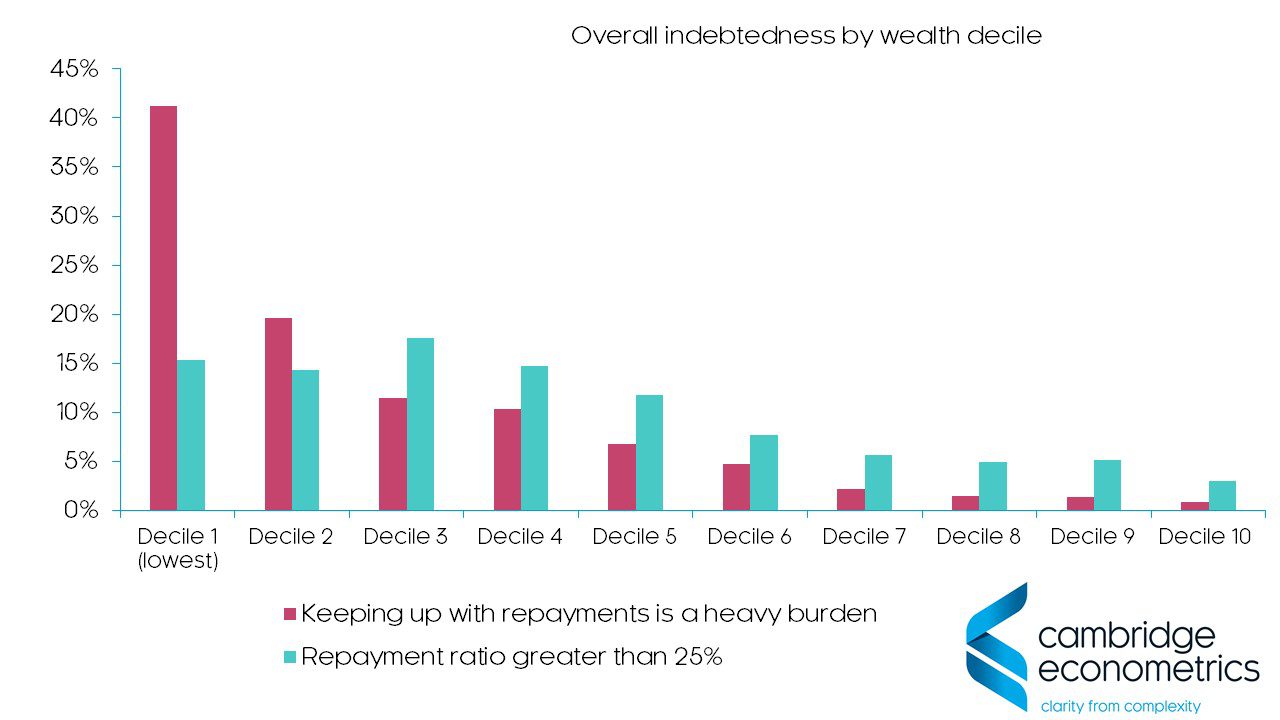
- The subjective debt measure is higher than the actual debt repayment ratio. I think this measure of subjective debt could be capturing a broader notion of over-indebtedness and could act as an early warning of future arrears – see Table 1.
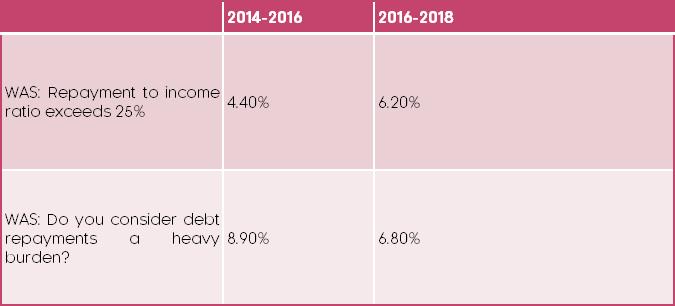
The Survey findings quickly showed the value of microdata in informing potential policy analysis with its combined focus on economic and social impact through the qualitative and quantitative indicators.
I would not have been able to gain such insights through looking at traditional aggregate statistics, demonstrating the merits of microdata in potentially aiding more targeted policy interventions.
Looking ahead
It will be fascinating to see what the 2018-20 round of WAS shows, given the current Covid-19 pandemic. Will over-indebtedness have increased markedly this year, what will policy makers do to address it? Here are my closing thoughts as we enter the second lockdown this year:
- With a depressed economic outlook since the start of the Covid-19 pandemic, the share of households considering debt repayment a heavy burden is likely to increase in the next round of the WAS due to be published in summer next year.
- As household incomes fall due to job losses, the share of over-indebted households as measured by the repayment to income ratio indicator will also likely increase, as existing debt repayment problems are exacerbated and some households that were previously financially sound start having problems.
- Households most likely to be over-indebted are also most vulnerable to Covid-19 related shocks, being in lower wealth deciles and often working in sectors such as retail and hospitality. As young people are more likely to be employed in those sectors, it is likely that there will be a disproportionate spike in over-indebtedness shares for age groups under 30.
- The Bank of England base rate cut will bolster resilience by lowering debt servicing costs (biggest effect on mortgages) and support the economic recovery.
- To solve the underlying over-indebtedness problem, a combination of improved financial literacy (given the high credit card debt), initiatives to raise productivity and wages, and regulation on precarious employment (zero-hour contracts) would lower over-indebtedness levels in the long run.
It is clear to me that microdata does indeed have a lot of value especially in the work that Cambridge Econometrics does drawing clarity out of the complex and often interconnected issues found across economy, society and the environment. I look forward to seeing how we can apply it better in our field of work, and how this can inform greater policy decision making especially during a global pandemic.
For more information on our latest news, follow Cambridge Econometrics on Twitter and LinkedIn