Explainer: What is scenario analysis and what can we learn from it?
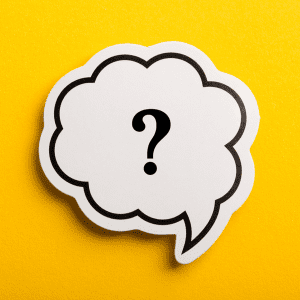
What is scenario analysis and why does it matter? Economic Modeller Rosie Hayward explains, commenting on a recent Twitter conversation on the purpose of mathematical modelling and using the concept of a spherical cat in a vacuum to illustrate.
Mathematical modelling entered the Twittersphere recently, when the chair of the Scientific Pandemic Influenza Group on Modelling (SPI-M), Graham Medley, attempted to explain to Fraser Nelson, the editor of The Spectator, why SAGE’s modelling results differed from real-world data.
Medley stressed that “scenarios are not predictions”, emphasising that they are made to illustrate possibilities and uncertainty in order to aid decision making. Nelson questioned why best-case scenarios were not also modelled, and Medley explained that scientists were not asked to model best-case scenarios, as in these cases no decisions would have to be made.
A wave of misunderstanding ensued, implying that worst-case scenarios were being deliberately predicted to ensure more restrictions, as seen in the resulting Spectator article . The fundamental purpose of the modelling was misrepresented, hitting close to home for those who work with E3ME, Cambridge Econometrics’ global economic model.
E3ME is a tool for scenario analysis. The analysis of how inputs to the model, such as a carbon tax policy decision, impact its outcomes, such as reduced CO2 emissions. These are hypothetical situations, and what is most likely to occur is impossible to predict.
This is not to say that experts are unable to make predictions or to confirm the certainty of some things in our future. It is vital that the difference between predictions, certainties, and the analysis of hypothetical scenarios are communicated clearly.
What is scenario analysis?
In the recent big-screen allegory for climate change, Don’t Look Up, scientists attempt to warn the world of a giant asteroid heading for Earth.
The speed, position, and trajectory of the asteroid are known, so the scientists can predict with certainty that it will hit the planet, although the oncoming disaster is painted as uncertain throughout the film, frustrating PhD candidate Kate Dibiasky. All the initial conditions are known here, the situation is not hypothetical in any way, and there are no blind spots; this is a calculation of what will happen.
Scenario analysis differs from this greatly.
Certain factors are always unknown, such as how quickly a virus may spread through a population, or whether policy makers will make a particular decision. Here, mathematical modelling is a useful tool for assessing what may be possible, not what is probable.
“The best model of a cat is a cat” – Dimitri Zenghelis
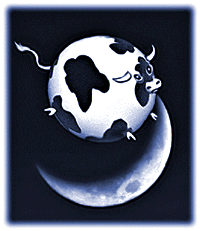
To honour this quote from a recent meeting with Dimitri Zenghelis, I decided to use spherical cats in a vacuum to illustrate my points here, rather than the proverbial cows. Can a spherical cat in a vacuum ever be a good model of a cat?
Let’s consider a cat leaping off a small tree (assuming it lands safely). We already know that cats are subject to gravity – we can count this as experimentally verified. Therefore, if we know all the initial conditions of the cat’s leap, we can make predictions about its trajectory with reasonable confidence.
If our question is simply, “when a cat leaps from a tree, what is the approximate time taken for it to land on the ground?”, then a model of a spherical cat in a vacuum will give us an answer close to that of more complicated and accurate models. Here, it is a good model of a cat.
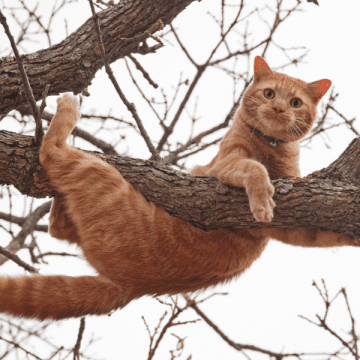
Complications arise when we ask how the cat falls.
Now we must account for the cat’s shape, any movements it makes, and any drag from the air. Our model becomes significantly more complicated, and the answer becomes significantly more dependent on the initial conditions. The cat will fall, but how it falls depends on many factors, and accounting for each one makes our model more complex.
A spherical cat in a vacuum would not be a good model of a cat here. Our simplifications are only problematic if they are inappropriate for the question being asked.
The application of simplifying assumptions which run counter to reality are highlighted by Cambridge Econometric’s former chief economist Hector Pollitt as a key flaw in many economic models.
“Scenarios are not predictions” – Graham Medley
Knowledge of classical physics would let us build an extremely realistic simulation of the leaping cat, but without full knowledge of all initial conditions this model could never be used to predict a real cat’s exact trajectory.
Instead, it is a tool which tells us about the generalised leaping cat, and the physics which governs them.
But if models cannot predict anything, what is the point?
Although we cannot predict the outcome of all future cat flights, we can ask how the height of its leap will affect the time taken for it to land, gaining insight into the potential outcomes of a cat leap; this is scenario analysis. Running our model for different leap heights cannot account for sudden gusts of wind, but it will illustrate possibilities and allow us to analyse the situation.
The outcome will be advice to all cats that leaping higher will result in them taking longer to land, when controlling for gusts of wind and other unforeseeable factors.
Mathematical modelling for scenario analysis is a powerful learning tool which helps us assess the impact of decisions, or differences in unforeseeable initial conditions, on a model’s results.
It allows us to learn how changes to initial conditions (policies for economics, bigger leaps for cats) will affect the model’s existing evidence-based relationships. The question which remains is whether the rules implemented in the model are a good reflection of the economy, or cat, it is applied to.
Can a spherical cat in a vacuum ever be a good model of a cat?
It depends on the model’s foundations and purpose. It is essential that economic models of the future are data driven, eliminating bias, and used responsibly with a full understanding of the boundaries and limitations, to ensure we can make the best decisions for our planet going forward.
Before the question of ‘what does this model tell us about the world’ is ever asked, we should first ask ‘what does the world tell us about our model’. From here, we can begin to learn.
Sign up to our newsletter today for the latest news, opinions and analysis from around the world.